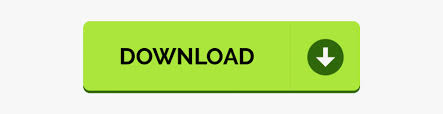

Will you sink or swim?įorbes Technology Council is an invitation-only community for world-class CIOs, CTOs and technology executives.Bryan Redmond, chief executive officer, Suntex Marinas (Suntex Marinas, Westrec Marinas, iStock) Higher employee satisfaction and retention. Greater creativity in problem-solving and innovation.Ĥ. Faster and more effective decision-making.Ģ. When that happens, organizations will find they are propelled forward by:ġ. Manufacturers - or any business - will be set apart by their ability to incorporate DQ and TQ into the hiring and training frameworks. This reality highlights the need for a generational shift in how and on what we train teams. Every line associate - not just team leaders - needs to interpret data as part of their daily job so that continuous improvement truly is continuous. The problem identification and solution process discussed above has moved from being infrequent to being recurrent thanks to Industry 4.0. Ultimately, as with anything, immersion and practice are the keys to learning a new way of working. There is no doubt that some people have innate abilities in data literacy and critical thinking that will make it easier for them to adapt to data-rich environments. It's less about the numbers and more about the domain knowledge, sleuthing, logic and people skills. In this process, one uses IQ, EQ and TQ - as solutions most often involve getting people to understand the issues and buy into solving them. In the lean world, this process is referred to as "PDCA" (plan, do, check, act). Some experimentation and problem-solving will follow before the results are implemented, tested and reported on. Is the issue impactful (frequency, repercussions of each occurrence)? If impactful, what is the best problem statement? Getting a crisp, accurate problem statement is half the solution.

Once an abnormality has been identified, the process of issue resolution immediately kicks off. With experience and training, these abilities can be acquired.
#Rising tide interactive cto linkedin how to#
In fact, there are three distributions that matter in their context, and one must determine what the basic descriptive statistic for these distributions are and how to apply these measures to determine if the processes they are responsible for are within control bounds. Practically, it is enough for one with a high DQ to know that not every dataset is a bell-shaped Gaussian. The pragmatist in me understands that is utopian. The geek in me might suggest that's possible only if one is trained in scientific thinking (i.e., able to use first principles to understand its distribution and the associated mathematics). As an example, it's necessary to understand the characteristics of the data presented to know if the behavior is abnormal. I have tried twice, at different times, to learn music and am now convinced that I don't have a musical bone in my body.) It is my belief that the abilities associated with data intelligence and thinking intelligence are most certainly learnable.įor example, consider data intelligence. My kids call me the lifelong student, as I firmly believe there is nothing that cannot be learned.
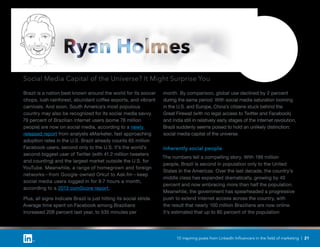
These skills are becoming more important as robots take on more of the physical labor and associates need to focus on higher-order production issues.īuilding a team with the skills needed in the digital age. In the simplest terms, the data intelligence quotients measure the first point: Does the person understand what is happening (e.g., what the problem is and what's causing it)? Similarly, the thinking intelligence quotient measures how well the person can deconstruct the problem, articulate a set of possible solutions, pick one and fix the problem using that approach. However, it's utterly useless if the person looking at the visual representation cannot interpret what behaviors the data represents or articulate what the problem is and how it might be fixed.

There are an increasing number of applications that focus on transforming - and then refining - data to create visual models for process monitoring, anomaly detection, root cause analysis and knowledge extraction.Īll of this innovation is helpful in turning numbers into charts and graphs that can be used at a glance to affirm that all is well or to uncover an issue that needs to be addressed. Manufacturing execution systems, manufacturing resources planning systems and enterprise resource planning systems are all sources of raw data and environments where data is transformed into insights. The benefits of applying these constructs.ĭQ and TQ in the context of what we're measuring.What organizations can do to foster the development and application of these skills.
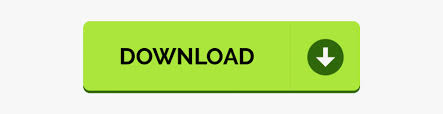